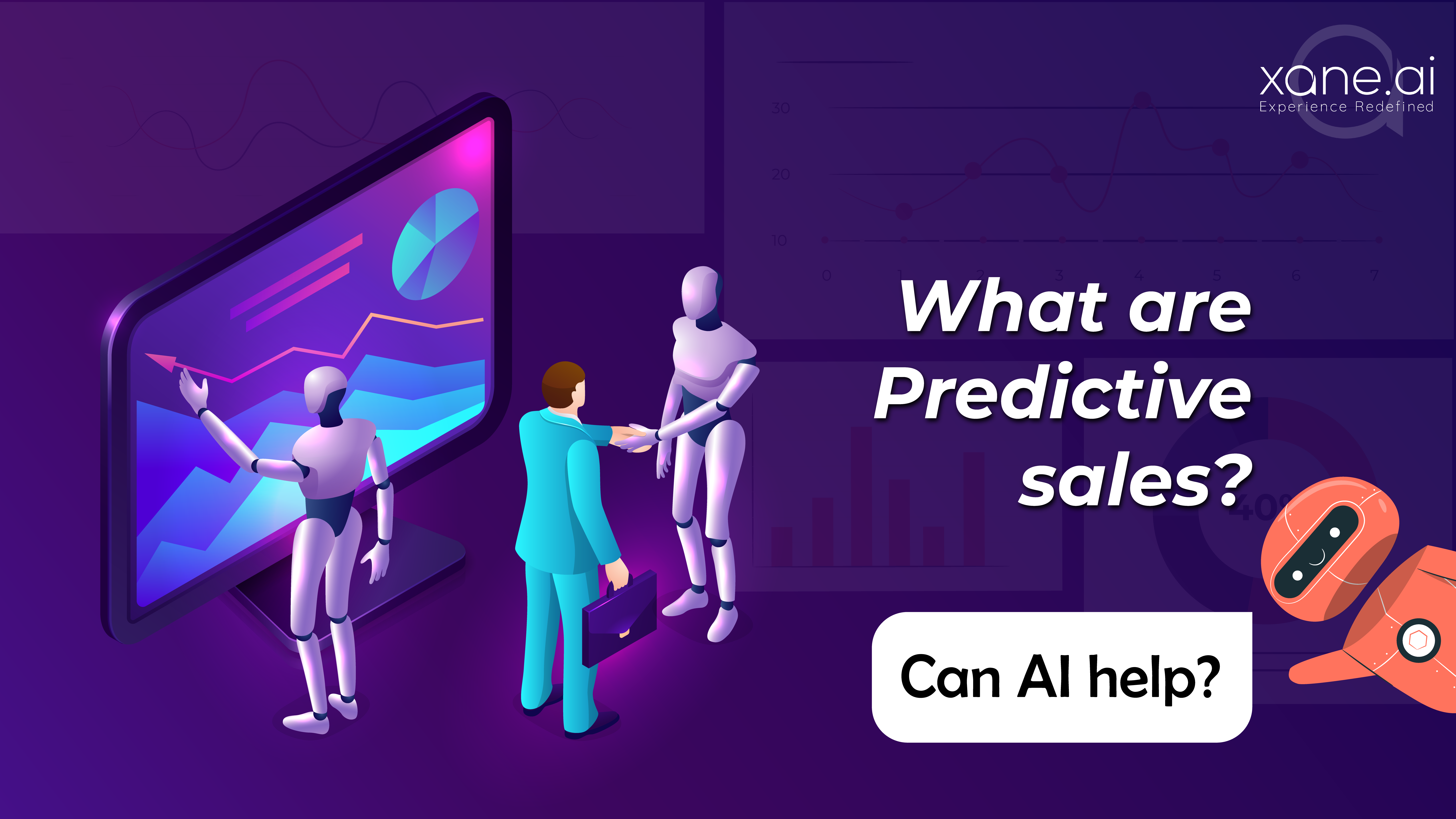
01 Sep What are Predictive Sales and How Can AI Help?
Blog by Oluwasegun Oke
The largely uncirculated victories and epic lack of uniformity between most targeted sales performance, and decreased ROI, increasingly factor in new AI interventions to optimize overall possible impacts, put away the menacing authority, increasing complexity, gigantic clout, and terrifying height of today’s commercially generated data. But don’t panic! AI needs more of this, knowing it is able to handle big data, function effectively. And what process can take up and convert such accumulations of partly unuseful data to a heap of gold? It is evidently models training, and it often precedes predictive sales, in generating customized marketing campaigns, much needed in advancing beyond every competition.
Big Data
Big data have long been around us before AI. It was the main reason experts embarked on a mission to invent it, in the first place. So without big data, AI wouldn’t have surfaced, let alone come into the reckoning. Indeed, big data coverage is expansive and somewhat intimidating. For this same, further development of newer models has taken place, to transform big data into useful information, and overwhelmingly manage all prospects in its accumulation successfully, which without AI, gained notoriety for frustrating data measurement, consumer understanding, and predictive sales.
Therefore, it just takes a second to figure out how in close proximity you are too big data. Moreover, have you considered, for example, how colossal and unfathomable, are the amount of data, handled on a daily basis, by big corporations, and government agencies? In order words, for every AI-driven natural language processing platform, used in converting document data to correlated digital formats, to extract quality insights about prospects, which can be must be successfully secured, to interpret historical marketing analytics, before engaging in predictive sales.
Models Training
A process powered by deep learning, which allows behavioral frameworks in complexly diverse big data to be studied, perfected and replicated in a new data (trained model). It is therefore used by commercial entities to learn and understand all behavioral aspects of different target audiences. However, in order to develop personalized products and services that can fit into specific trends of potential customers, certain AI-intensive instruments, must be installed. And the more rooted, structured, relevant, and varied the available big data, so needed to carry out every step of this model training, the more the usefulness or expert level of the trained algorithm model, in that particular field. Actionable insights have proven over the years, to be critical in following to a logical conclusion, every collected customer interaction session, using bots, chatbots, automated email promos, CRM and AI-coordinated social media platforms.
AI and Sales Prediction
Actionable insights have proven over the years, to be critical in following to a logical conclusion, every collected customer interaction session, using bots, chatbots, automated email promos, CRM, and AI-coordinated social media platforms. And this is crucial in meeting the sophisticated scope of sales prediction and providing further information to be used on subsequent marketing performance.
Furthermore, AI plays a major role in automating every marketing analytics process, which offers rich metrics about such previous performance standings, which can be interpreted to forecast future sales, by introducing human intelligence.
With AI, more potential clients are able to be kept abreast of the latest development, about additional products and services. With this process in place, constant audience engagement becomes evident, to further cement a long-lasting relationship. With this process in place, constant audience engagement becomes evident, to further cement a long-lasting relationship.
Without AI, financial recklessness would gain prominence and threaten corporate entities’ financial clouts, inciting a state of chaos and data to disintegrate. A situation where a gigantic amount of data would be wasting away, or perhaps seeking for answers, with zero prospects of ever converting them to useful insights.
While getting the best of big data has its most intriguing perspective, which can only be properly harnessed and transformed into useful insights by AI-driven technology, to collect, interpret, and gain correlated intelligence and insights about potential customers, by interpreting generated marketing analytics reports.
Besides, AI eradicates complexities in human errors, and automates real-time, clear-cut decision-making processes, to develop proper sales plans, and stimulate growth. Apparently, potential customers are only interested in subscribing to customized products and services. This leads us to introduce, a case study, where the same AI-predictive technology is used by Netflix, Youtube, and Apple Siri, to recommend different entertainment-packed materials and other interests alike, for users to get full value and be committed to a long-time relationship.
And as it turns out, a brand’s constant connection with its target audience, improves in a major way, its chances of converting potential customers, and turning around such a company’s fortunes.
Significance of Data Mining and Algorithm Models Fields
And even when a pool of data sets consist of varying elements, and most times invalid information. This prompts us once more, to apply AI to contract all unused spaces, around such a piece of information, or better still extract relevant segments, in order to recognize different entities. And in a relatively shorter span, arrived at better data, well structured, informative, uniformed, useful data, that is only synonymous to a particular customer. Notably, AI ensures that such a data sales field would be eventually streamlined to meet specific customer needs. Let’s discuss an example below, to illustrate how data sales fields collected via an email potentially works:
- First name of Buyer
- Last name of Buyer
- Address of Buyer
- Buyer’s Telephone number
- Buyer’s Email
- Buyer’s Address
- Buyer’s T-shirt or Shirt Size
- Buyer’s Debit or Credit Card Type
- The exact time the order was made
- Pieces or units ordered
- Previous Times such Customers made similar Purchases
- The Name of the Products
- The Specific Product Type (for instance “T-shirt or Shirt” )
More parameters can be added to the above list, to extend models’ frameworks, sensitivity, and proactiveness to any length, by enriching each field, with critical elements, and desired flexibility. This impacts on the particular trained model, the providence (relevant in the future), that only AI-powered tools possess and ensure that each model is not only well versed, but predictive, and able to handle a large number of queries (big data).
As such, the extracted field is clearly well defined and accurately fine-tuned, to avoid ambiguity and the generation of invalid algorithms models. But if this happens, restraining such models is indispensable. This ability, that AI possesses to spontaneously recognize and identify even lines, and boundaries between useful and error-ridden data sets give it a critical place in solving complex data issues.
Inadvertently, having the same level of informative fields, on different products, every season, would be more than enough to generate a quality lead, to help understand, separately assign, and streamline widespread customer behavioral patterns, on offered products. And what next, is to further advance the fully automated AI-driven tools to transform all accumulated fields into useful insights, then marketing analytics reports. Now, the interpretation of these analytics is what eventually provides sales leaders and marketing teams, with the right marketing strategies, that are applicable promoting to specified product s.
While on good terms, more marketing stakeholders have left behind all archaic techniques and measurement practices — including traditional marketing analytics reports — to embrace AI predictive sales technology. With this, next marketing campaigns are better streamlined to fit specific customer behaviors, thereby improving sales and upgrading customer experience.