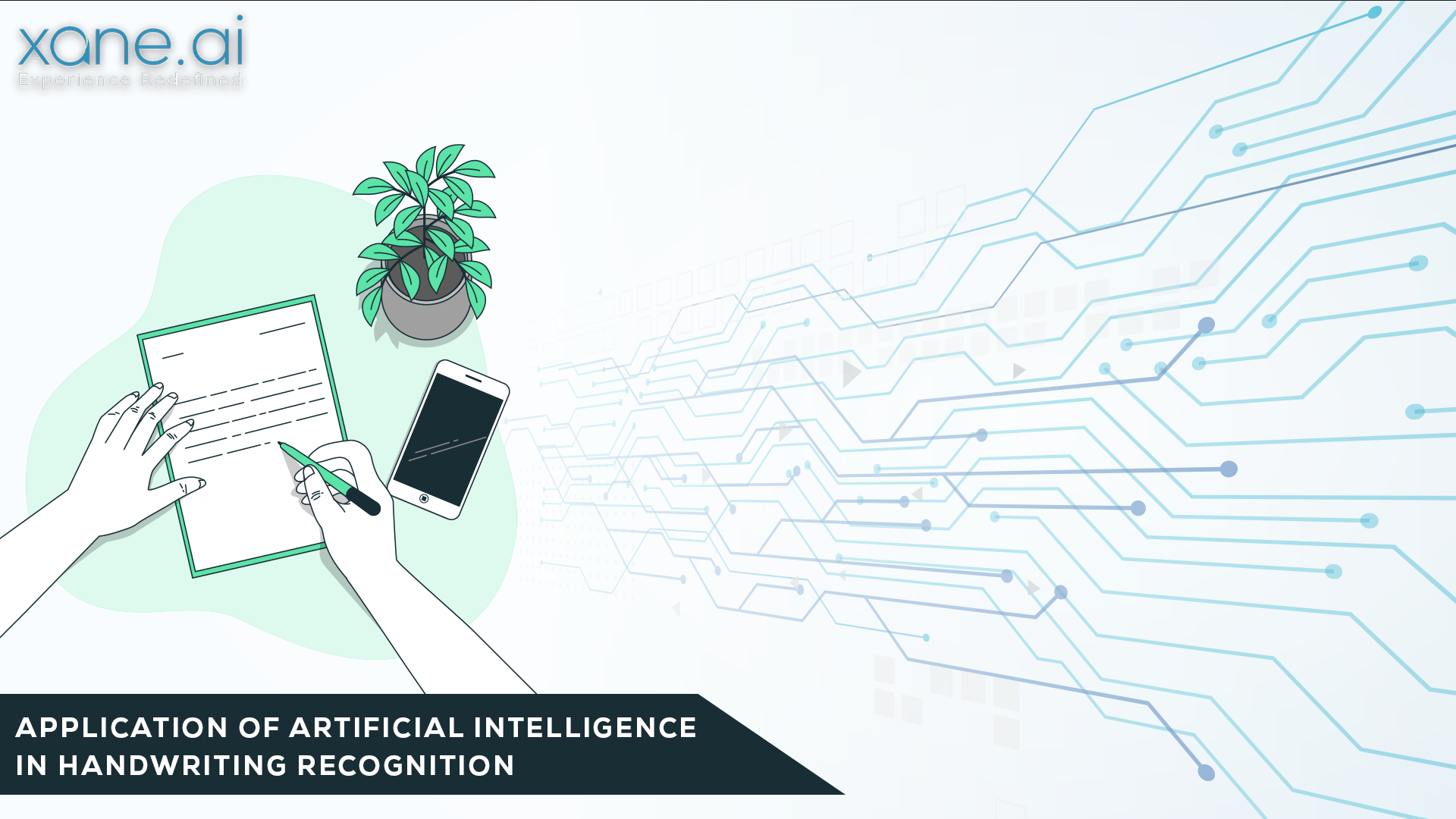
18 Jan The Application of Artificial Intelligence in Handwriting Recognition
Blog by Oluwasegun Oke
In a world where datasets constantly undergo further experimental research, harnessed for new capabilities, incorporated into a variety of technologies, and positioned for the future. These facts interestingly put forward how much Optical Character Recognition (OCR) is a rapidly growing project, by cutting down on business workforce and man-hour, for staggering return on investment. And to dissect the epic journey from traditional marketing to digital, we recognize and emphasize the replacement of olden days monotonous advertisement on Print Media, as opposed to Online Marketing, of which principles thrive on simplicity and creativity of such campaigns to attract customers and marketplaces.
Thus OCR presents us with the unique opportunity to further ride higher on our main goal of operating within the digital space by employing, for instance, bookkeeping, files processing, attendance notes, etcetera, which are often activities from traditional organization structures, by successfully converting these inputs into a Machine-readable text, through Computer Vision, Machine learning, and likewise Artificial Neural Network, for a high degree of achievable quality, character solidity, and completely adjustable document text resolution.
WHAT IS OPTICAL CHARACTER RECOGNITION?
It basically means conversion of Handwritten Text Character into Machine-readable text, from both table and word documents. Therefore it scans the documents for already programmed (Recognizable) characters and makes exact replications of such in a digital format. This is how businesses reach their global audience, with colossal profits that continually remind us about the need to shift focus to Intelligence Character Recognition (Handwriting Recognition), which has exponentially revolutionized interface attributes of fast-tracking business activities, between combining traditional workplaces with the digital space.
CHALLENGES FACED BY OPTICAL CHARACTER RECOGNITION
Scanning of documents for text does come with its shortcomings. Because many scientists, engineers, artists, business owners, researchers, and so on, do not have the best handwriting, this creates a drawback as the Recognition Machine works to extract the right characters and compares them to those on its stored database system. Regardless, it has been widely validated and diversely corroborated that long reliance on computer keyboards, among other factors, instigate major causes of deteriorated handwriting.
CORRECTION OF OPTICAL CHARACTER RECOGNITION CHALLENGES
With the aforementioned setbacks, it becomes essential to create across platforms, absolute computer accuracy to recognize characters, and extract successfully, data from paper documents. Nevertheless, in order to process and override these anomalies, Deep learning and Artificial Neural networks become essential. Deep learning empowers by initiating the capability for computers to learn and understand previous varieties of obtainable patterns of human handwriting over time, and effortlessly decipher the real-time meaning of letters, digits, and signs (characters) on such source paper documents.
On the other hand, Artificial Neural Network works on the basis of the collection of nodes of which interact (communicate), exchange and process filtered data information line the human brain. And as such, create more or less separated layers of interconnected nodes (up to 150), and therefore offer an advanced level of data storage, executing successfully comparison of document characters with its database system for detecting and correction of errors, while correctly extracting even badly written text, coupled with synapses across nodes which act as hidden integrated layers, to form a complex network of superior and equally adaptive brain quality, to resolve extensive handwriting recognition problems. In theory, each neural represent a neuron in biology, because the scope of Artificial Neural Network was developed based on processes of handwritten recognition and diffusion of neurotransmitters, found in the human brain – an elastic mass that can multiply over and adapt to excessive reception or rush of information, by validation and absorption of further complexity and increases in the intensity of information communicated across layers, to process an unlimited number of data
TECHNOLOGY PLATFORMS THAT OFFER CHARACTER RECOGNITION SOLUTIONS
Following current subscriber demand that has created over $8.7 billion market value chain worldwide, the extraction of paper document data by Handwritten Text Recognition Solutions and Optical Character Recognition Services, such as Xane AI is offering, have broken myriads of interface boundaries, present superior PSA, and integrate personalization by incorporating transparency and objectivity through a legal framework, and today advanced emerging from within strategically located service centers, a chatbot inclusive website, and major digital social media channels, to reach, connect and enlarge the interest and projection of its well-positioned clientele while providing further fully automated decision making solutions.
Similarly, its AI-powered handwritten recognition mechanism proudly boasts of maximally extracting and achieving 95% use cases accuracy, with its efficient Computer Vision and Natural Language Processing. Therefore, maximally capturing the intended volume of documents in record time, without backing out on customer satisfaction, with its accelerated extraction business documents coverage on warranty claims, invoices, cheques, bank statements, etc. As it centrally fetches KYC information from documents, coupled with up to date processing then automatic number plate recognition, with which it professionally leads the way and continually engages user experience with reducing the cost to service.
CAREER FIELDS AND USE CASES
HEALTHCARE AND PHARMACEUTICAL
Many pharmaceutical and healthcare patients’ biometric database systems are often converted format of handwritten documents. It further cements OCR and HCR technology as indeed hands-on in practice. And increasingly, processing and keeping record cases, where patients prescription digitization and Handwriting Recognition Software, found in their powered equipment room, optimize lifesaving efforts and promote informed accelerated engagement on duty as well as the opportunity to handle millions of petabytes of medical PDFs daily.
INSURANCE
No insurance institution dare look away and diverge from the work ethic that places them solely on fully automated means of the filling of claims document, as to ignore this may lead to serious backlash and complete stalemate of their handwriting recognition system, and in turn, their official engagement. This becomes imperative, given that certain insurance companies receive more than 20 million documents daily.
BANK
Handwritten Text Recognition System dissolves queues in banks, shorten man-hours, safeguard return on investment, and mitigates against straining of the eyes and fading away of vital written document characters, due to excessive mishandling, in the course of duty, to ascertain the authenticity of check signatures and dates, which is essential to fast-tracking the processing and integration of valuable front desk questions to fully automated answers, as compiled and deciphered from their central system, which operates in compliance to handwriting recognition solutions.
ONLINE LIBRARY
Handwriting Recognition sparks back to life the symbolism and noble causes from inspirational medieval days, valuable postcards, research studies, and so on. Judging by the humongous size of historical knowledge being stored through continual transfer from text-inscribed documents to digital format, which allows users to query and browse varieties of information after entities have been indexed.
HANDWRITING RECOGNITION METHODS
Online Method: It basically traces out demarcation as imprinted along with the character pattern, with each stroke of the stylus or pen, being used on the digital application device, to write text with a high degree of accuracy for recognition and information transfer.
Offline Method: it does not show any pattern of each stroke, involves in its creation, but practically displays the finally written text, for recognition, while leaving behind background noise of its paper medium. In essence, no digital stylus pen is put to use.
HOW TO SOLVE OFFLINE TEXT RECOGNITION PROBLEMS
Deep learning has accurately fractured and optimized the quality and efficiency of handwritten recognition, as obtained through its superior scalable extraction for any language. As opposed to machine learning of which performance is limited to how much text is preprocessed to identify inflection points, loops, aspect ratio, etcetera, which passes through, for instance, HMM for possible results. But in the case of Machine learning, preprocessed extraction differs extensively from one language to the other, and it’s therefore not scalable.